The scheduling activities in a flexible flow shop system
Sep 1, 2023 5:30:10 PM - 10 min read
Nicola La Palombara
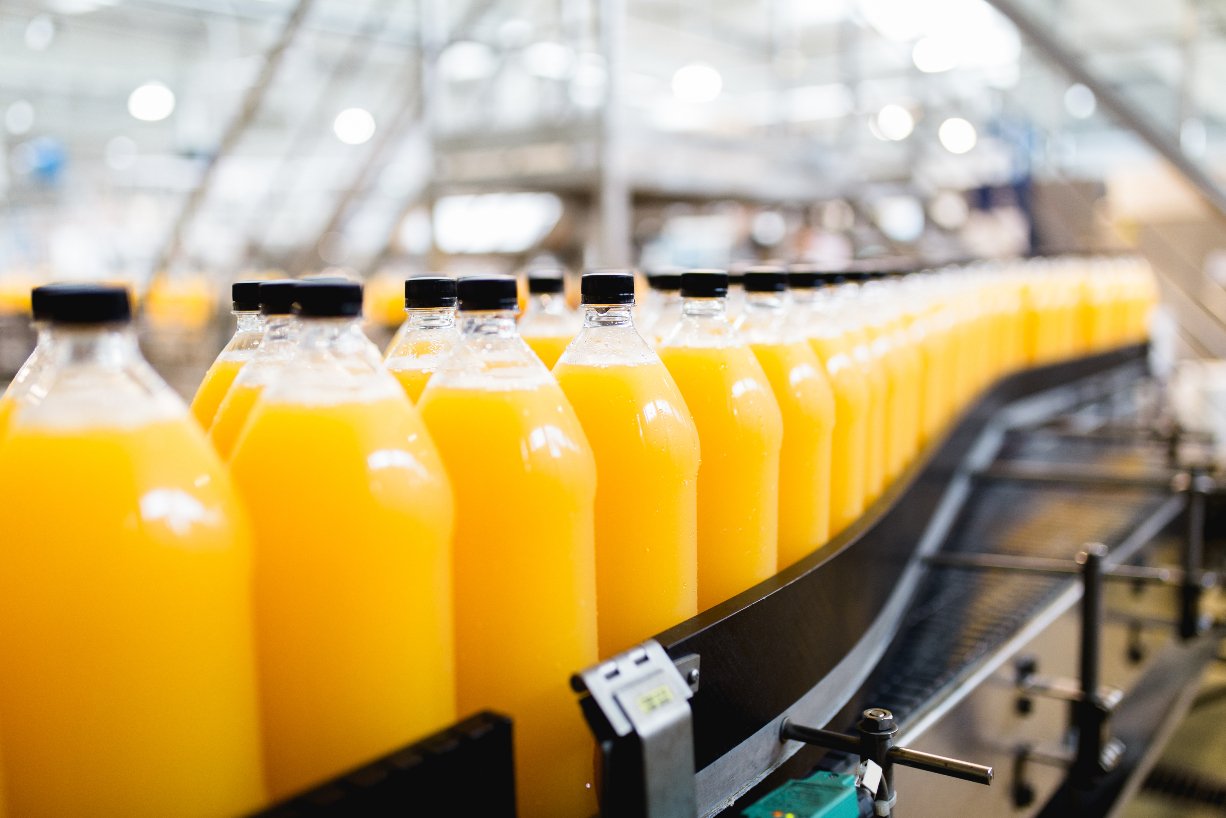
Scheduling function in a production system represents the last decision-making phase previous to the execution of production plans. Considering all the overlapping stages of production planning and control (PPC) activities (see Fig. 1), scheduling problems relate to the allocation of limited resources to activities, over time, to fulfill the requirements established in the planning phase [1].
Scheduling role is to find a solution that describes what to produce, on which resource (where), at which time (when), with the aim to satisfy customer demands, as well as minimize costs. Moreover, when unexpected events compromise the solution's effectiveness, so-called rescheduling activities are triggered to update the plan. Some triggers of rescheduling are the arrival of new jobs, breaks, and repairs of machines, delivery delays, etc.
The hybrid flow shop scheduling problem
Scheduling problems are strongly influenced by the shop floor layout where operations occur. In this article we will analyze the hybrid flow-shop (FFS) scheduling problem, commonly known as flexible flow-shop (FFS), which arise from the combination of other two important machine scheduling problem [2]:
-
The flow shop scheduling problem where a series of different machines is arranged in multiple stages with only one machine at each stage
-
The parallel machines scheduling problem where there is a series of identical machines, all at the same stage.
Based on this consideration, an FFS scheduling problem is related to a group of parallel machines arranged into several stages in series (see Fig. 2). At each stage, there are more or less identical machines in parallel. Each job has not to be processed at each stage, and it can ignore one or more stages. Usually, each machine can process at most one job at a time.
Many variants of the problem exist, which we can mainly classify based on three factors [3]:
a. Shop configuration
This axis distinguishes problems based on the number of stages in the shop, the number of parallel machines in each stage, and possible differences among them.
b. Constraints and assumptions
This axis defines the rules and limits of the processes i.e., machine processing and interaction with jobs. For example, basic models often assume infinite buffer capacity and any job can be processed by any machine in a stage. However, sometimes, a job can be processed only in a subset of machines, due to technical or physical reasons. In real environments we always have limited buffers and machines' set-up time cannot be considered all equal.
c. The considered objective function
The objective is typically to minimize the make span, which implicitly attempts to maximize the utilization of the system. In general, theoretical research has focused on models with a single objective. In the real world, there are several objectives, but their respective weights may vary over time and may even depend on the context.
The hybrid flow shop in real production system
The FFS corresponds to a widely adopted workshop organization in the industry, particularly in sectors such as cosmetics, agri-food, and pharmaceuticals. In these industries, the generic process consists of a series of operations that follow a precise order (preparation, manufacturing, and packaging), with resources arranged in parallel at each stage.
In this type of system, workshops are organized and sized to ensure a continuous flow. Raw materials are introduced at the beginning of the chain and move from one operation to another with minimal intermediate storage.
Furthermore, workshops must be sized to handle seasonal peaks without causing delays, and in many cases, they must be flexible enough to handle different types of raw materials and/or finished products. From this perspective, the FFS is perfectly adaptable to variations in production volumes due to its design, which allows for an increase or decrease in the production system's capacity by opening or closing parallel resources at certain stages of the process.
The FFS organization is particularly well-suited for mass production of standardized or moderately diversified products. Without altering the production sequence, diversity is often achieved by varying recipes and/or formats. Cleaning and format change operations, therefore, need to be managed in conjunction with production operations.
Solution approaches
Given its complexity and importance in real applications, the FFS problem has been intensively studied and many solutions techniques have been developed.
Optimal resolution approaches provide the best results, but are impractical for even modestly sized problems. Heuristics are efficient but not always effective, often scheduling the jobs for the first stage and using the same sequence of processes throughout all stages. Artificial intelligence approaches find good solutions, escaping from local optimal, but these approaches are hard to construct and most of the algorithms are problem specific.
In addition, recent innovations brought about by Industry 4.0 foster the development of two very interesting streams of research [4] to tackle scheduling-related problems
-
The management of real-time information is highly desired by the industry
-
The decentralization of the decision-making process (see article on cyber-physical system)
The possibility to find valuable solutions is increasingly dependent on the ability to capture and use the large amount of data generated on the different aspects of the manufacturing setting. The design of the whole data acquisition and processing system is also very critical to capturing the frequent changes that occur at the operational level. The aim is to be able to react effectively to unexpected events that may compromise or even invalidate the solution found.
Real-world vs. theoretical model
The complexity and volatility of the production data considered is perhaps the greatest conceptual barrier separating the world of scientific research from real production systems. It is important to consider the real world is unpredictable and dynamic, making difficult the adaptability of operational research approaches. In fact, real-world flexible flow shop scheduling problems are usually very different from the mathematical models studied by researchers:
-
Theoretical models address problems with a set of jobs known in advance
In practice, frequent disturbances modify the assumption on which the current plane is based, forcing a rescheduling. Developing a schedule that is robust in facing unexpected events is advantageous to minimize the changes in the existing schedules.
-
Mathematical models always consider simplified management of production resources.
As already pointed out, is very optimistic considering all machines behave in the same way with total availability and the possibility of storing products in buffers with infinite size. Mathematical models do not consider the assignment of extra shifts and overtime, which are possible options for planners.
Hence, a concentrated effort is still required to model most real-life situations in order to effectively apply recent methodologies to complex FFS problems.
Future perspectives
Despite the many differences discussed, the insights developed from theoretical research have proven to be useful in the development of the algorithmic framework for numerous real-world scheduling systems. The most critical aspect remains the relatively high frequency of random events which often cause schedule changes during its execution that may not be worth spending high computation time to find an optimal solution that can be compromised in a few hours.
Few results can be found on robust scheduling in FFS: we need to develop models and methods which provide, not only efficient overall production performance but also reactive, to face the frequent set of unforeseen events. An interesting area of research could be the development of so-called hybrid approaches, which combine predictive (offline) and dynamic (online) scheduling techniques.
In the absence of a perfectly satisfactory resolution method, major efforts should be directed towards: Exploiting the possibilities offered by new I4.0 technologies, such as cyber-physical systems.
-
The ability to control, compute and communicate the huge amount of data in production chains is the challenge cyber-physical systems try to meet. With a combination of digital computation and physical control, CPS is designed to support real-time applications and promote the decentralization of the decision-making process, enabling reactivity based on locally available information.
-
Developing ergonomic scheduling assistance solutions with simulation and collaborative working capabilities
It is fundamental to develop decision support tools to simplify the planners' working environment. For example, being able to display several sets of information at the same time and giving the possibility to change the current status or current information. In addition, simulation tools are crucial to obtain alternative solutions in parallel, instead of a single one that may not be efficient.
In summary, effective scheduling of production systems in general, and FFS configurations in particular, relies more on responsiveness and anticipation than on the exact resolution of the underlying mathematical problem. The development of cyber-physical systems combined with the emergence of new scheduling software solutions contributes to enhancing schedulers' ability to anticipate and react, leading to effective trade-offs between validity and performance.
BIBLIOGRAPHY[1] M. L. Pinedo, Scheduling. Cham: Springer International Publishing, 2016. [2] H. Wang, ‘Flexible flow shop scheduling: optimum, heuristics and artificial intelligence solutions’, Expert Syst., vol. 22, no. 2, pp. 78–85, May 2005.
[3] R. Ruiz and J. A. Vázquez-Rodríguez, ‘The hybrid flow shop scheduling problem’,Eur. J. Oper. Res., vol. 205, no. 1, pp. 1–18, Aug. 2010.
[4] D. A. Rossit, F. Tohmé, and M. Frutos, ‘Production planning and scheduling in Cyber-Physical Production Systems: a review’, Int. J. Comput. Integr. Manuf., vol. 32, no. 4–5, pp. 385–395, May 2019.